Foundational artificial intelligence may still be in its infancy, but it’s already being used to help bring new varieties with better traits to farmers’ fields. Tech Farmer takes a look at some of the AI innovations that are set to speed up the breeding pipeline.
Imagine having a brain that never gets tired and retains every last piece of information it has ever learned. A brain that can see patterns in data and make complex calculations in millionths of a second.
These are just some of the capabilities artificial intelligence (AI) is already bringing to our everyday lives as groups of AI technologies form systems capable of performing tasks that traditionally have required human intelligence (known as foundational AI).
The computational power of artificial intelligence (AI) has a very natural fit with plant breeding as nature tends to follow mathematical rules. Gregor Mendel is regarded as the ‘father of genetics’ because he worked out so much about how traits are passed from one generation to the next using just maths.
From his experiments in peas, Mendel developed a mathematical formula that explained the frequency with which each trait appeared. He also observed dominant and recessive traits despite having no knowledge of DNA or genes.
Modern plant breeding still follows Mendelian processes, but the advent of molecular-based marker-assisted technologies to identify genes has sped up the process considerably compared with phenotypic screening alone. Now advances in technology, such as cloud computing and increased processing power, are moving speed breeding up another gear.
For Bayer, the journey began over a decade ago when the company began to integrate data science into its breeding programmes to help optimise the process.
“We started with a simple idea and that was to take ‘the breeder’s equation’ and work out how to apply machine learning to optimise every term in that equation”, explains Phani Chavali, who leads Bayer’s US data science team in plant breeding.
The breeder’s equation is used to predict breeding outcomes by indicating how strong the response to selection will be as a result of the additive genetic variance within a trait, and the selection applied to that variation. Phani explains that by using AI and machine learning models, Bayer has become better at predicting outcomes, essentially speeding up selection for genetic gain.
“We have developed genomic selection, which is a machine learning model that uses genotyping data to predict the performance of different genetic material. And using that information, we can advance varieties in the breeding pipeline without actually testing them in the field,” he explains.
“We also use machine learning to select new breeding crosses, and to advance the progeny from these new crosses into subsequent years.”
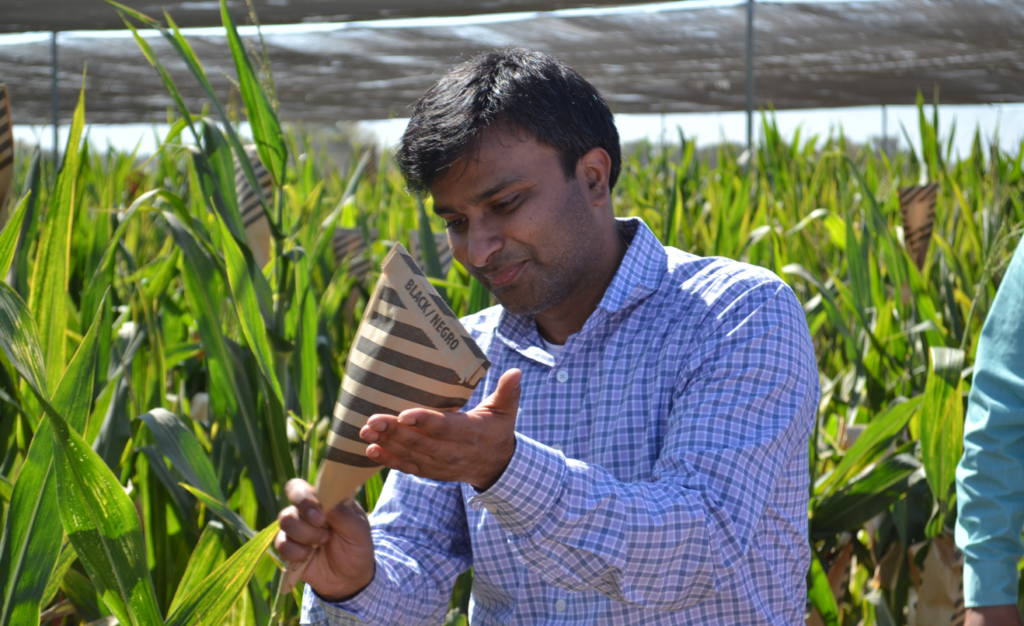
This AI assistant is helping breeders select the most promising candidates and it relies on cloud-based algorithms built on a foundation of roughly 1.7 trillion calculations. These models have enabled a dramatic shift in the scale and speed of the breeding pipeline, and over the past 15 years it has saved the company from running around 8000ha of field trials.
“On average, 5.2M predictions run each day to support the breeding programme. That’s a huge number and the amount of data we collect to feed the algorithms is over one petabyte [there are 1,024TB in one petabyte],” says Phani.
Advances in AI capabilities have enabled Bayer to embark on a new phase in its plant breeding in the past few years, with the aim of leaving the Mendelian processes of probability behind.
“The idea behind this ‘precision breeding’ strategy is to make a shift from selecting the best to designing the best,” he says.
Computer vision
Another plant breeding company is combining other dimensions of AI – machine learning and computer vision – with autonomous robots.
At its US breeding facility in Illinois, KWS is using AI to collect vast amounts of phenotypic data that hasn’t been humanly possible before. Here, the TerraSentia robot can be found trundling noisily up and down the alleyways that run between the trial plots at the huge wheat variety trials site, snapping photos as it goes.
The phenotyping robot was developed by EarthSense, an agritech start-up based at the State’s university. The company’s co-founder and chief technology officer, Girish Chowdhary, describes some of the challenges they faced when creating the TerraSentia robot.
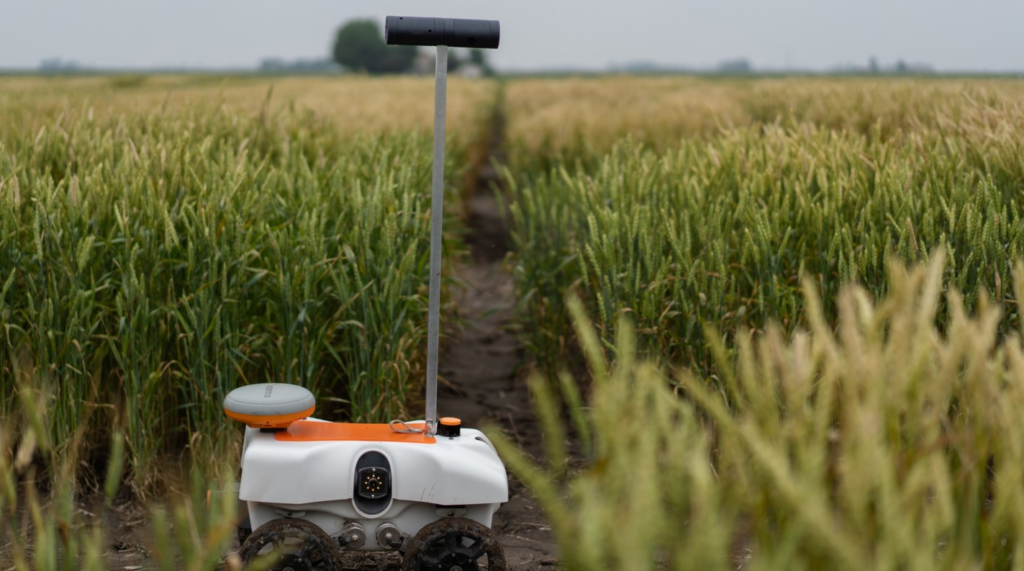
“It’s moving in a very noisy and uncertain environment. We’ve tried to account for that by building a ruggedised robot so it can work well and collect good data under these conditions.
“Cameras can move around because the surface the robot is working on isn’t very stable, and they can also be blown about by the wind. We’ve incorporated allowances in our machine learning algorithms to account for any variability in the data due to these issues.”
The robot routinely does the legwork that humans would do, but it’s the AI, or computer vision that’s currently learning to interpret the images the robot is capturing that could be the game-changer.
“The idea behind this ‘precision breeding’ strategy is to make a shift from selecting the best to designing the best.
Girish describes the machine learning process as creating a large set of data labelled by humans. The data is fed to machines and its software generates knowledge from experience by means of repetition, identifying patterns in the data that relate to traits, diseases, or growth stage, for example.
Its neural network is then able to create a new mathematical model, an algorithm, as it ‘learns’. Once the artificial intelligence has obtained enough knowledge from humans, it uses it to compare new images and identify phenotypic expressions. In the case of the robot, it evaluates the pictures of plants without the need for human assistance.
KWS wheat breeder Mark Christoper explains how the robotic trials are going. “The fact that the robot can operate continuously and independently means it’s able to collect data on more material than we’ve been able to in the past. This will allow us to make more informed selection decisions, especially in our younger generation breeding nursery where we have hundreds of thousands of individual rows and it’s just not feasible for humans to collect all the data.”
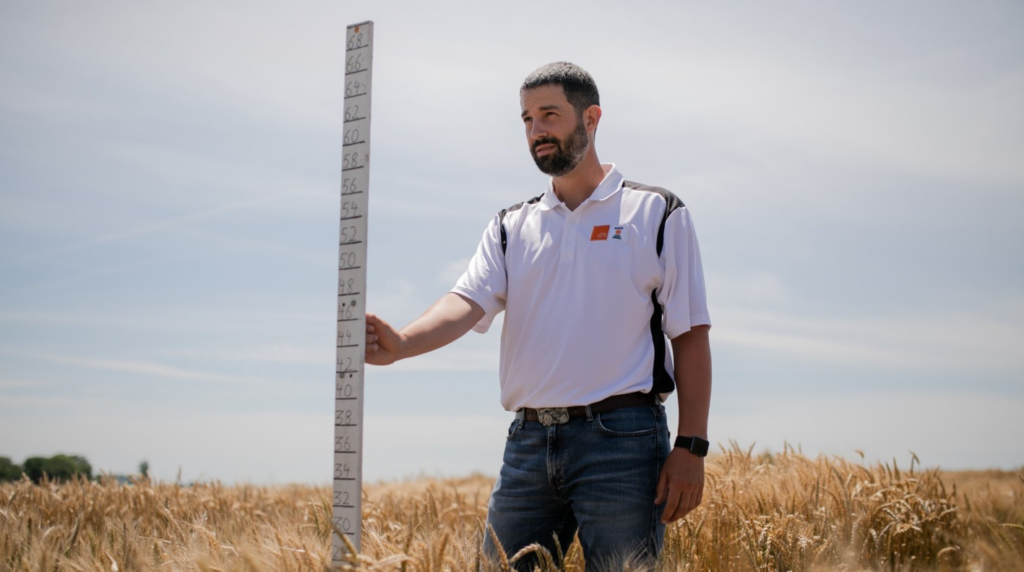
So far, the trials work has shown that the AI model is able to identify traits such as awn type and heading date using plant images from the trial field. It’s also highly accurate, with results showing that the AI detects emerged ears with 96% reliability and that it can identify whether an ear is completely awned, or not, 92% of the time.
More recently the data collected has been expanded to look at additional traits such as plant height and disease severity.
“Comparing robotics and humans, they each have their own strengths. The robot will be very good at providing very objective, high-quality data for specific traits, but the human is required for making those subjective advancement decisions. And then there are certain things that the breeder’s eye is required for,” he adds.
It’s an important point that humans are not being replaced in the breeding process, but instead AI is augmenting their decision-making, adds Mark. “Data provided by the robot will add precision to the decisions that we make, and this will help us produce better varieties.”
Predicting germination
There are other tasks where AI and machine learning could lend plant breeders a helping hand – one of those is germination testing. Recently, researchers have taught a new tool – SeedGerm – how to do it using machine-learning-driven image analysis.
The innovative machine has been developed to perform the process in a semi-automated way and is the result of a collaboration between the Earlham Institute, the John Innes Centre, Syngenta and NIAB.
Carmel O’Neill, research assistant in the Penfield Group at John Innes Centre explains that currently most seed germination is recorded manually. “Compared with this, SeedGerm presents fast, accurate, high-throughput screening and will be of major interest to crop seed production companies and research programs screening large germplasm collections.”
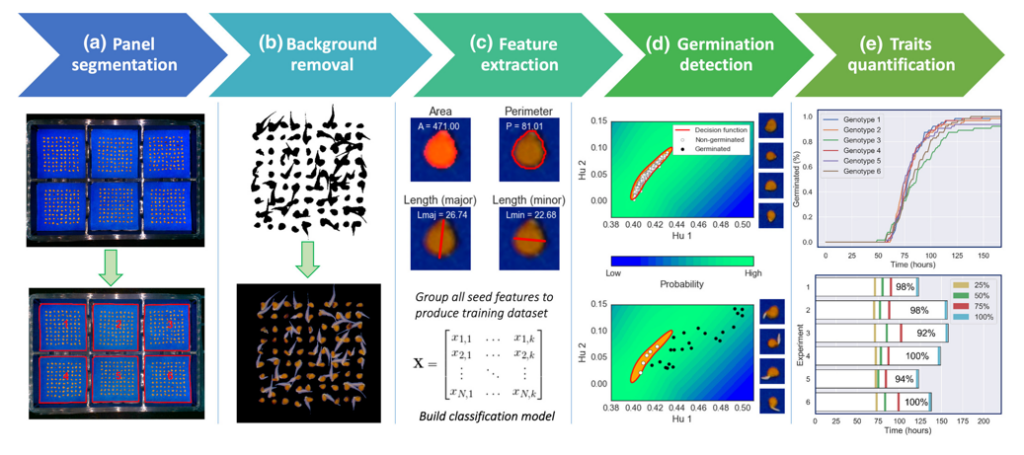
SeedGerm uses a cabinet equipped with cameras which take photographs throughout the germination process, documenting each stage from imbibition (seeds taking up water) through to the emergence of the root, and further changes in the newly growing plant.
Supervised machine learning is used to automatically determine how germination is progressing by comparing images. Algorithms can be trained to predict how likely it is that a seed has germinated based on measurements extracted from an image that relate to the seed’s size, shape, and colour.
Seed germination experts from Syngenta have confirmed the effectiveness of SeedGerm for measuring germination rate and seedling health across five major crop species, including tomato and oilseed rape, opening the way for SeedGerm to replace manual seed scoring.
In addition, the power of SeedGerm to measure phenotypic changes over time has further novel applications in crop improvement research. Many of the characteristics that can be measured help to estimate performance in the field in terms of canopy closure, weed suppression and predicted yield.
RNA Sequencing
While AI is already bringing evolution to breeding programmes, the main focus in plant breeding is on DNA and the genes within it. But a revolution may be brewing that’s focussing on sequencing RNA and the importance of gene expression.
In simple terms, genotyping data identifies the DNA sequence and provides an indication of potential (the presence or absence of a gene that are predictive for a desirable trait), whereas RNA sequencing (RNA-seq) gives biological meaning (or what will likely happen) by quantifying how much the gene will be expressed and other coregulated genes.
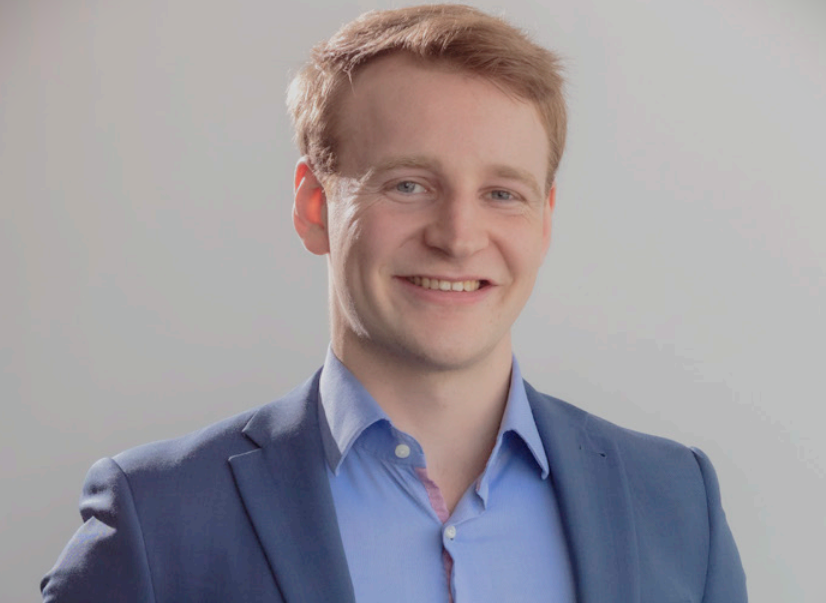
“This is significant because it highlights a limitation when only using DNA-based methods for marker-assisted breeding,” says Dr Joshua Colmer, cofounder and CEO of agritech startup TraitSeq. “Analysing DNA alone doesn’t present the full picture, especially in cases where traits are complex – meaning they’re affected by multiple genes.”
Many of the traits that plant breeders are looking for are complex in nature, such as drought tolerance or nutrient use efficiency, and consequently these characteristics are hard to breed for.
TraitSeq’s platform technology uses bespoke machine learning methods and bioinformatics tools to identify biomarkers. These are then used to train phenotypic prediction models for complex traits in crop plants. Using its proprietary RNA-seq analysis methods, the company aims to help breeders by predicting phenotypic outcomes for complex traits to help inform selection decisions and optimise breeding programmes.
TraitSeq’s IP was developed by Joshua during his PhD at the Earlham Institute, where he used machine learning to develop models that were able to successfully predict outcomes from RNA-seq data to a high level of accuracy – including the prediction of turnip mosaic virus infection, human cancer subtype classification, and the diagnosis of COVID-19 infection.
It’s this computational capability that could bring a new dimension to plant breeding. The technology has the ability to accurately predict measurable targets that relate to changes in phenotype, physiology, or metabolism under varying environmental conditions.
“We could predict the field performance of a trait based on glasshouse trials, for example. This is also an aspect that crop protection companies are interested in to help them screen potential new active ingredients – identifying those that are likely to fail in the field so that they can be removed from the innovation pipeline without running costly field trials,” explains Joshua.
The same can be done in variety trials which could significantly speed up the breeding process. “What is exciting is that where traits were previously difficult or expensive to measure in field trials, TraitSeq can be used as a predictive phenotyping tool. As sequencing prices continue to fall, it will become a more cost-effective way to phenotype those traits and implement them into a breeding program.
“The types of traits this is applicable to aren’t limited to yield, disease resistance or nutrient deficiency. It could be applicable to quality traits like protein content or water absorption for baking quality,” he adds.
Another advantage of RNA-seq technology is it reveals how genes regulate each other. “With a sufficiently large data set, you’ll be able to identify genes that regulate the expression of other genes. And by manipulating the expression of transcription factors, it might be possible to predict how that could affect other traits [such as yield] downstream.”
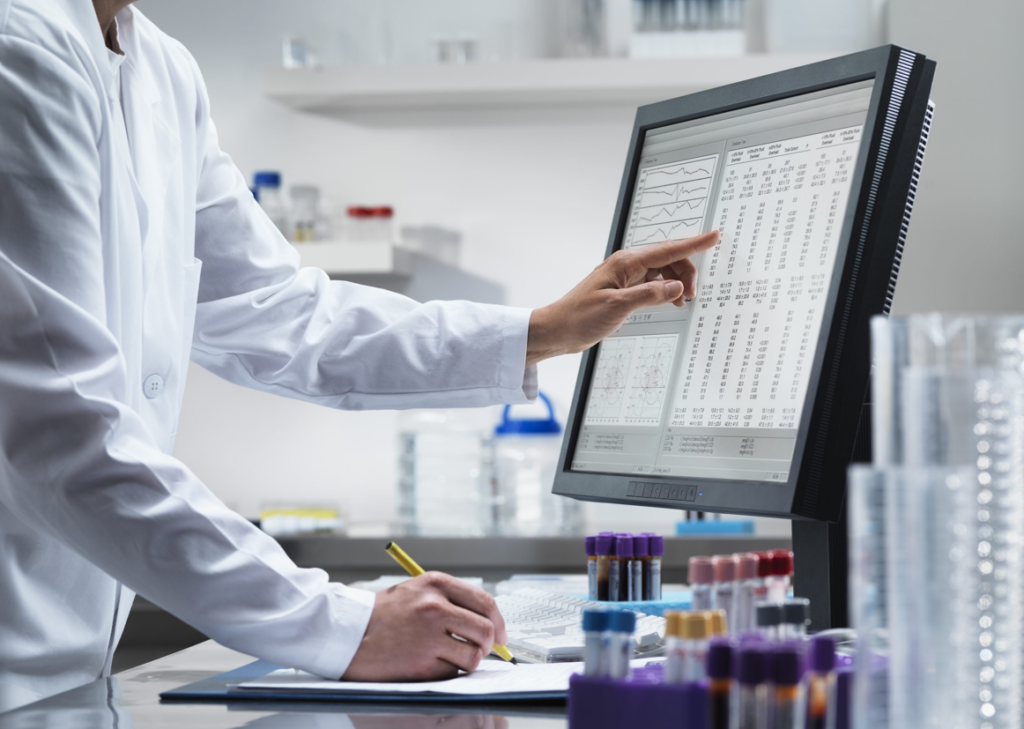
It’s still early days for the company that has recently spun out of the Earlham Institute and received funding from UKRI’s Innovation to Commercialisation of University Research (ICURe) pilot programme. This backing, together with substantial funding from Anglia Innovation Partnership and Innovate UK, is enabling TraitSeq to work with companies on pilot projects.
The biggest hurdle to commercialisation at the moment is the current cost of RNA-seq compared with genotyping, reflects Joshua. “Breeding companies have invested significant resources into marker-assisted breeding methods and developing genotyping platforms. The assays they use cost on the scale of pence per datapoint, whereas RNA-seq is around £200 per sample. It’s a huge difference in cost so we have to demonstrate there’s a lot of added value in RNA-seq technology, particularly in its ability to enable the prediction of complex traits.”
Joshua predicts the cost of the testing will come down considerably. “It’s not unreasonable to expect RNA-seq to be £30-50 per sample in three to five years. However, we can use qPCR as another means of quantifying gene expression, which is a much cheaper method. Once our algorithms identify gene expression markers for a complex trait from RNA-seq data, we can develop a qPCR assay to test for it which brings the cost down to around £10 per sample.
“All the while we are constantly developing our computational platform for RNA-seq data analysis. So once the technology becomes cheaper and more widely adopted, we aim to be the go-to solution for companies to obtain meaningful insights from the big data they will be generating,” adds Joshua.
And there’s no doubt that big data, in all of its guises, is informing food production throughout the value chain. Yet the advancement of AI isn’t without concerns.
In a cautionary tale, The Matrix hit the big screen 25 years ago. Set in 2199, the film depicts humanity enslaved by a cyber intelligence that it had created – ‘the machines’ had taken over. The reality in 2024 is that much of AI use is pretty mundane but the potential for misuse remains. So to protect against this, UNESCO set out an ethics framework for AI systems in 2021, one of which is that human supervision is vital.
Perhaps the ingenuity of humans in creating AI capabilities will be invaluable in meeting the world’s climate and food security challenges. As long as ‘the machines’ won’t be making the actual decisions…
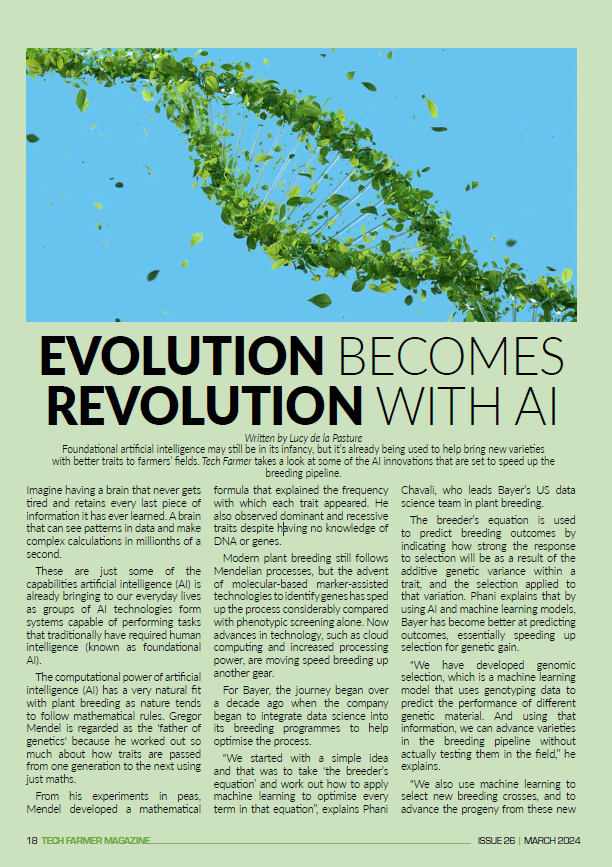
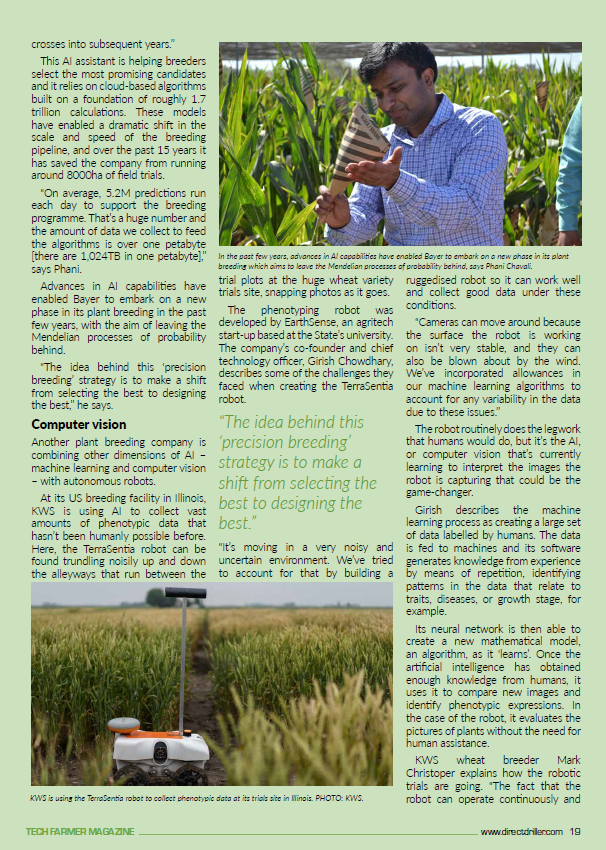
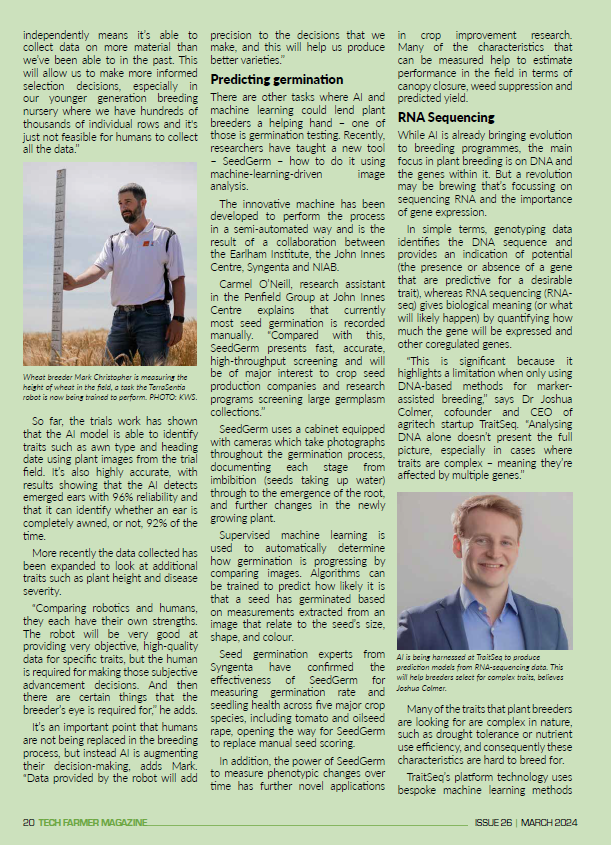
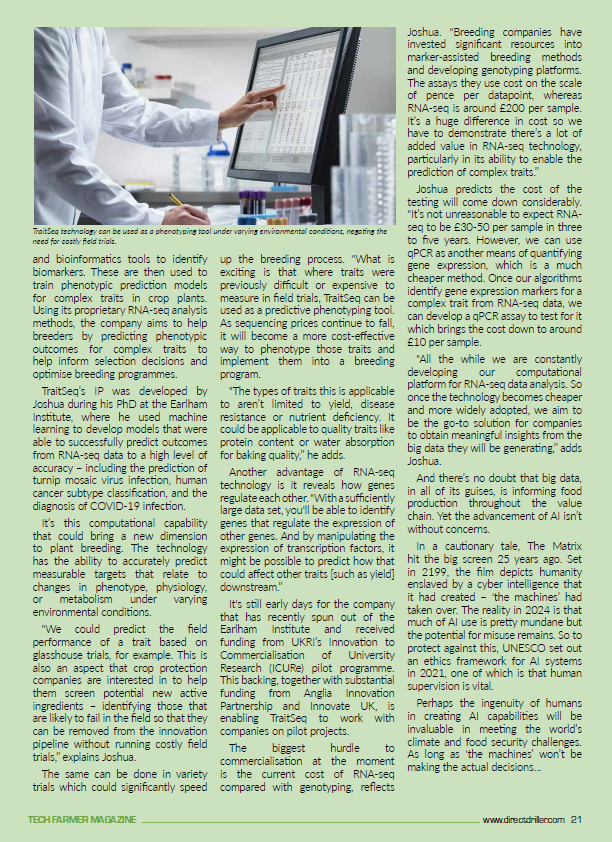