Unlocking the potential of big data can lead to smarter farming, but there are complex interactions and challenges to navigate.
In the dynamic landscape of modern agriculture, the integration of big data technologies has revolutionized the way farmers operate and make decisions. Smart farming, fuelled by the inseparable relationship between production and consumption of data, presents both opportunities and challenges that necessitate a deeper understanding of its intricate dynamics. Addressing critical questions raised by farmers and practitioners is paramount to unlocking the full potential (and potential farm level profitability) of big data in agriculture.
The dual role of farmers: users and co-producers of data
In the realm of smart farming, farmers assume a multifaceted role as both consumers and co-producers of data. They harness the insights gleaned from data analysis to address real-time challenges while simultaneously shaping the information landscape through their management practices. This duality presents a unique challenge to the institutionalisation of big data, as every decision made by a farmer influences the quantity and quality of the data generated. Understanding the nuanced interplay between farmers’ actions and data production is essential for fostering effective integration of big data into agricultural practices.
Big data isn’t bad when it’s helping you grow better yields, but just sending farm data down the supply chain carries with it risks.
Navigating complexity: farmers’ adaptation to evolving data
Unlike traditional tools, big data are characterised by continuous evolution, with each action generating new insights and altering the nature of the tool itself. Farmers must adapt to this ever-changing landscape by developing new knowledge and skills to effectively harness the potential of big data. However, the increasing complexity of data analytics poses a significant challenge, raising questions about farmers’ capacity to comprehend and utilize complex insights. Balancing the need for sophisticated data analysis with practical decision-making processes is crucial for enhancing the effectiveness of big data in agriculture.
Quality assurance in big data analytics
The exponential growth of data in agriculture raises concerns about data quality and reliability. While the sheer volume of data may seem promising, bigger does not always equate to better. Biases and inaccuracies introduced during data-handling processes can compromise the integrity of insights derived from analytics. Moreover, the transformation of raw data into actionable information adds another layer of complexity, influencing the accuracy and interpretability of results. Addressing the quantitative and qualitative aspects of data transformation is essential for ensuring the reliability of information provided to farmers.
Empowering farmers’ decision-making
Big data analytics are often touted as decision-making tools that guide farmers towards optimal solutions. However, the extent to which data inform decision-making processes varies, with farmers interpreting and utilising insights in diverse ways. While data-driven solutions offer specific pathways for action, they may also constrain farmers’ creativity and experimentation. Understanding the nuanced relationship between farmers and big data is essential for designing strategies that empower rather than restrict decision-making autonomy.
Ownership, privacy, and data governance
The collaborative nature of big data poses challenges regarding ownership and privacy rights. Unlike tangible assets like tractors, data lack clear ownership, leading to uncertainties surrounding data exchange and customisation. Farmers’ reluctance to share data stems from concerns about privacy and the potential misuse of their information by external actors. Establishing transparent data governance frameworks that safeguard farmers’ interests while facilitating data sharing is crucial for fostering active engagement and trust within the agricultural community.
Motivations and community dynamics
Exploring farmers’ motivations and goals sheds light on the underlying factors driving their adoption and use of big data. Farmers’ actions are influenced by a complex interplay of economic, social, and psychological factors, highlighting the importance of understanding individual and collective motivations. Moreover, the dynamics within agricultural communities shape the adoption and utilisation of big data, with diverse actors contributing to value creation and innovation. Collaborative efforts that integrate resources and expertise are essential for realizing the full potential of big data in agriculture.
Rethinking value creation and institutional arrangements
The distribution of power and value within agricultural communities is shaped by investments in technology, expertise, and resources. While big data providers play a pivotal role in data collection and analysis, farmers’ active engagement in value creation is essential for fostering sustainable agricultural practices. Collaborative value-creation frameworks that transcend traditional hierarchies empower all stakeholders to contribute meaningfully to the agricultural ecosystem. Moreover, establishing clear rules and norms governing data use and exchange is vital for ensuring equitable participation and fostering trust among community members.
In conclusion, the integration of big data holds immense promise for revolutionizing agriculture and addressing global food security challenges. However, navigating the complexities of smart farming requires a holistic understanding of the interactions between farmers, technology, and the broader agricultural community. By addressing key questions and challenges, stakeholders can work towards harnessing the transformative potential of big data to create a more sustainable and resilient agricultural future.
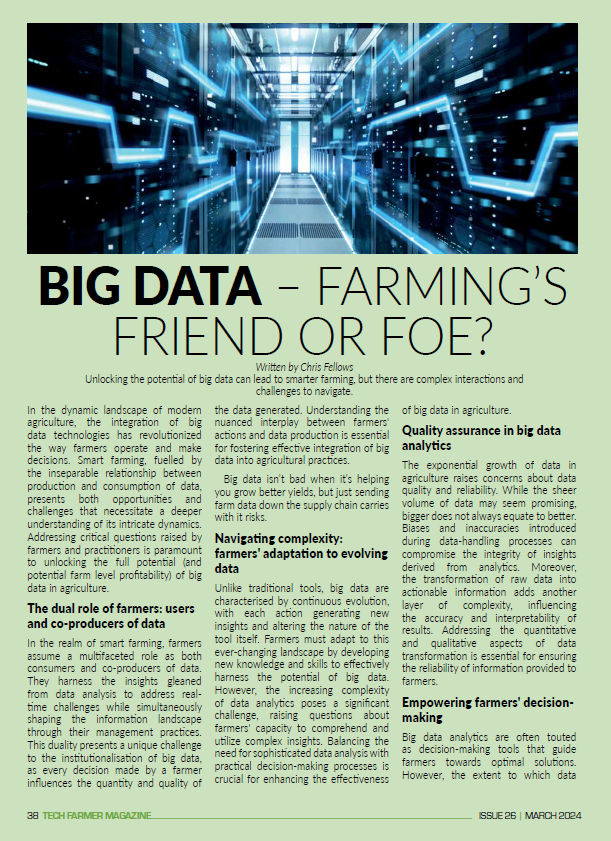
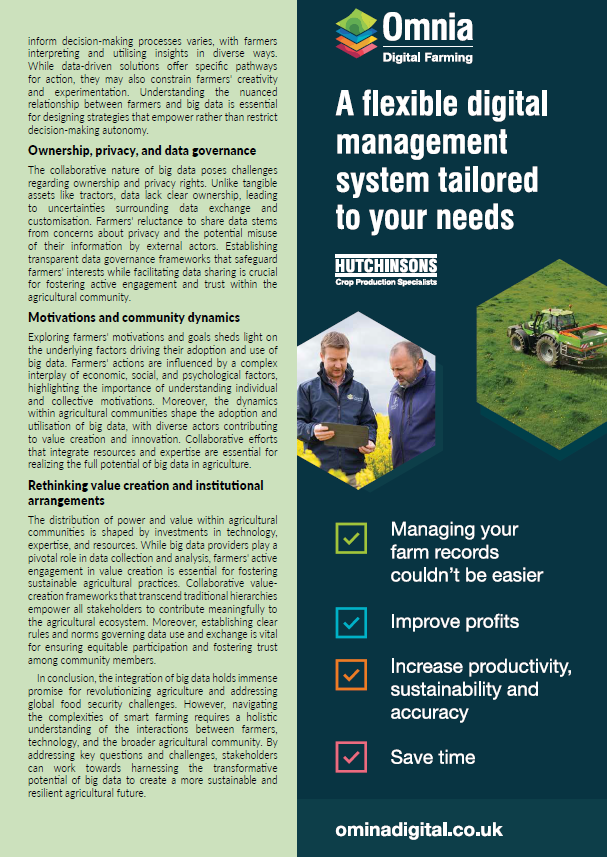